Boosting Monocular Depth Estimation to High Resolution
Simon Fraser University, 2022
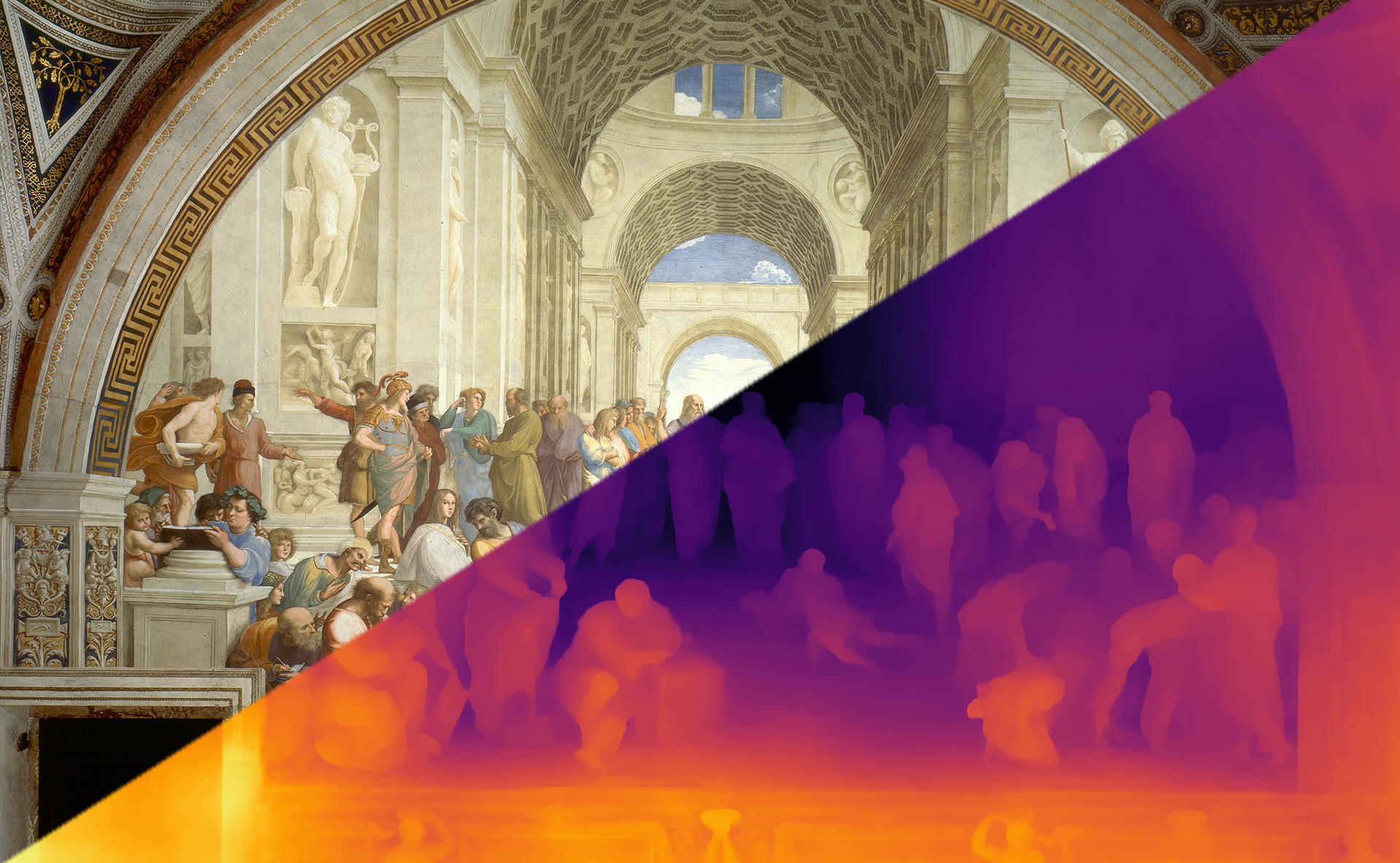
Depth maps generated for Fresco by Raphael in the Vatican City known as School of Athens by our method. This result was featured by Nature in their Best Science Images of the Month - August 2021 selection.
Abstract
Convolutional neural networks have shown a remarkable ability to estimate depth from a single image. However, the estimated depth maps are low resolution due to network structure and hardware limitations, only showing the overall scene structure and lacking fine details, which limits their applicability. We demonstrate that there is a trade-off between the consistency of the scene structure and the high-frequency details concerning input content and resolution. Building upon this duality, we present a double estimation framework to improve the depth estimation of the whole image and a patch selection step to add more local details. Our approach obtains multi-megapixel depth estimations with sharp details by merging estimations at different resolutions based on image content. A key strength of our approach is that we can employ any off-the-shelf pre-trained CNN-based monocular depth estimation model without requiring further finetuning.
Dissertation
![]() |
Video Presentation
BibTeX
author={Seyed Mahdi Hosseini Miangoleh},
title={Boosting Monocular Depth Estimation to High Resolution},
year={2022},
school={Simon Fraser University},
}
Publications in the context of this thesis