Metric Monocular Reconstruction through Ordinal Depth
Simon Fraser University, 2022
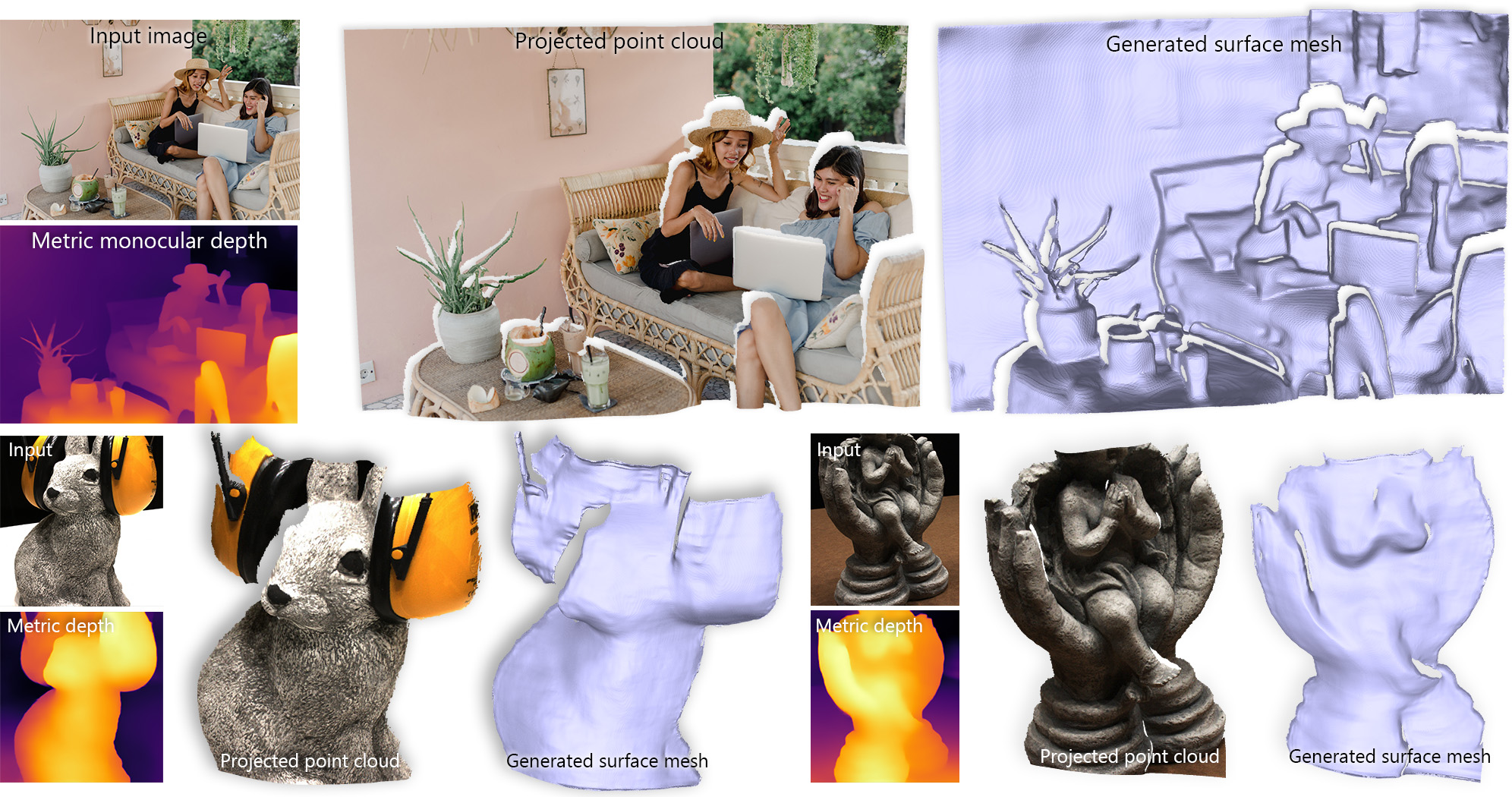
Abstract
Training a single network for high resolution and geometrically consistent monocular depth estimation is challenging due to varying scene complexities in the real world. To address this, we present a dual depth estimation setup to decompose the estimations into ordinal and metric depth. The goal of ordinal depth estimation is to leverage novel ordinal losses with relaxed geometric constraints to model local and global ordinal relations for capturing better high-frequency depth details and scene structure. However, ordinal depth inherently lacks geometric structure, and to resolve this, we introduce a metric depth estimation method to enforce geometric constraints on the prior ordinal depth estimations. The estimated scaleinvariant metric depth achieves high resolution and is geometrically consistent in generating meaningful 3D point cloud representation for scene reconstruction. We demonstrate the effectiveness of our ordinal and metric networks by performing zero-shot and in-the-wild depth evaluations with state-of-the-art depth estimation networks.
Dissertation
![]() |
Video Presentation
BibTeX
author={Mahesh Kumar Krishna Reddy},
title={Metric Monocular Reconstruction through Ordinal Depth},
year={2022},
school={Simon Fraser University},
}
Publications in the context of this thesis