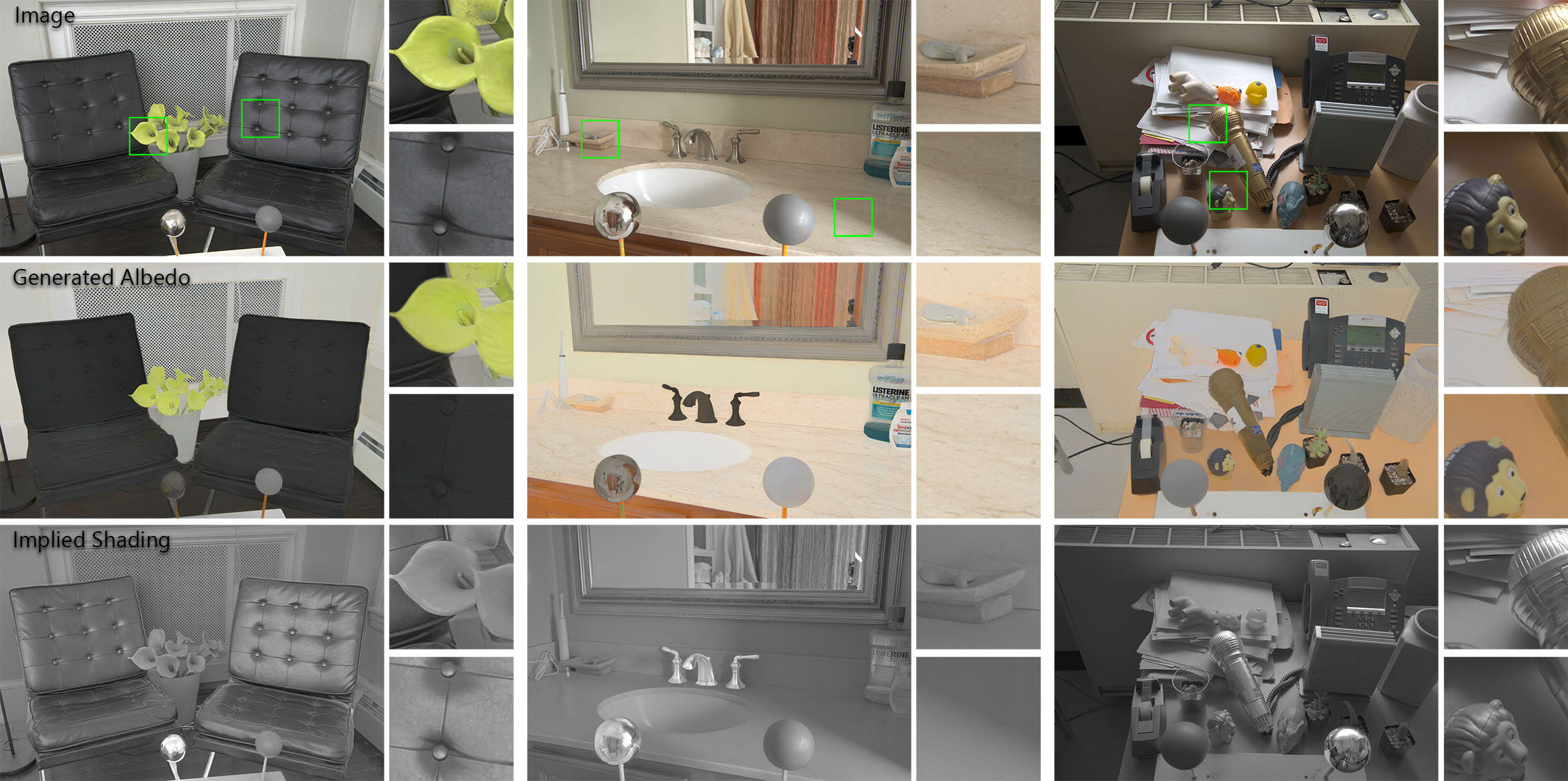
We generate dense pseudo-ground truth albedo and shading maps for the scenes presented in the Multi-Illumination Dataset by Murmann et al.
We present the first dense, real-world, and large-scale dataset for intrinsic image decomposition. Our dataset was derived from the Multi-Illumination Dataset by Murmann et al. and consists of 1000 scenes under 25 different illuminations each, with 1000 unique albedo maps and 25.000 image - RGB shading pairs.
Paper
The Dataset
BibTeX
If you use our dataset in your scientific work, please cite our paper and the publication behind the Multi-Illumination Dataset.
author={Chris Careaga and Ya\u{g}{\i}z Aksoy},
title={Intrinsic Image Decomposition via Ordinal Shading},
journal={ACM Trans. Graph.},
year={2023},
}
author={Lukas Murmann and Michael Gharbi and Miika Aittala and Fredo Durand},
title={A Multi-Illumination Dataset of Indoor Object Appearance},
booktitle={Proc. ICCV},
year={2019},
}
License
This dataset is shared under CC BY-NC-SA 4.0 for research purposes only. You can freely use it for scientific publications and include them in figures.
The methodology employed to generate this dataset is safeguarded under intellectual property protection. For inquiries regarding licensing opportunities, kindly reach out to SFU Technology Licensing Office <tlo_dir ατ sfu δøτ ca> and Yağız Aksoy <yagiz ατ sfu δøτ ca>.
![]() |
Intrinsic Image Decomposition via Ordinal Shading
Provisional Patent Application, 2023
|