A Dataset of Flash and Ambient Illumination Pairs from the Crowd
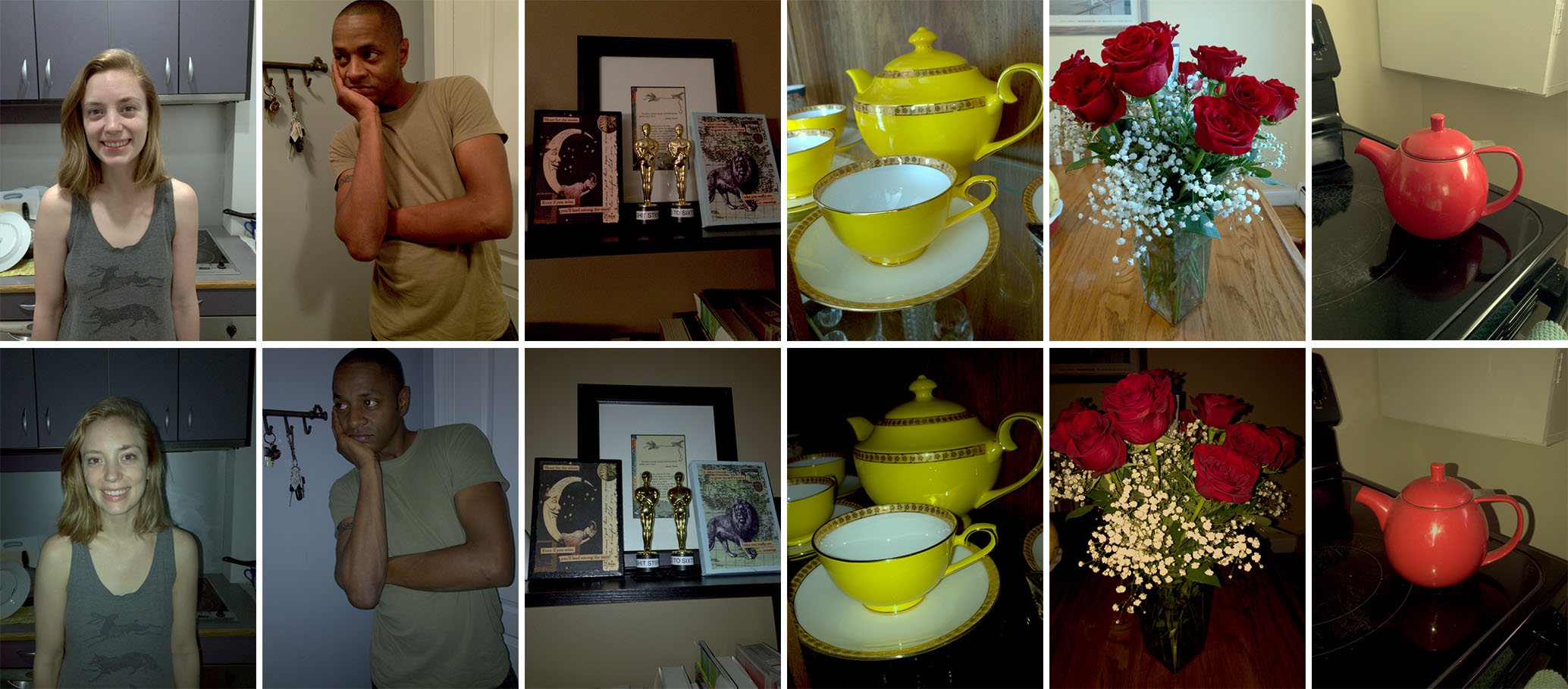
We introduce a diverse dataset of thousands of photograph pairs with flash-only and ambient-only illuminations, collected via crowdsourcing.
Abstract
Illumination is a critical element of photography and is essential for many computer vision tasks. Flash light is unique in the sense that it is a widely available tool for easily manipulating the scene illumination. We present a dataset of thousands of ambient and flash illumination pairs to enable studying flash photography and other applications that can benefit from having separate illuminations. Different than the typical use of crowdsourcing in generating computer vision datasets, we make use of the crowd to directly take the photographs that make up our dataset. As a result, our dataset covers a wide variety of scenes captured by many casual photographers. We detail the advantages and challenges of our approach to crowdsourcing as well as the computational effort to generate completely separate flash illuminations from the ambient light in an uncontrolled setup. We present a brief examination of illumination decomposition, a challenging and underconstrained problem in flash photography, to demonstrate the use of our dataset in a data-driven approach.
The Dataset
![]() |
The Flash and Ambient Illuminations Dataset (FAID) consists of aligned flash-only and ambient-only illumination pairs captured with mobile devices by many crowd-workers participated in our collection effort.
This dataset accompanies our ECCV 2018 paper.
@INPROCEEDINGS{flashambient,
author={Ya\u{g}{\i}z Aksoy and Changil Kim and Petr Kellnhofer and Sylvain Paris and Mohamed Elgharib and Marc Pollefeys and Wojciech Matusik}, booktitle={Proc. ECCV}, title={A Dataset of Flash and Ambient Illumination Pairs from the Crowd}, year={2018}, } |
Manuscript
![]() |
![]() |
BibTeX
author={Ya\u{g}{\i}z Aksoy and Changil Kim and Petr Kellnhofer and Sylvain Paris and Mohamed Elgharib and Marc Pollefeys and Wojciech Matusik},
booktitle={Proc. ECCV},
title={A Dataset of Flash and Ambient Illumination Pairs from the Crowd},
year={2018},
}
Related Publications